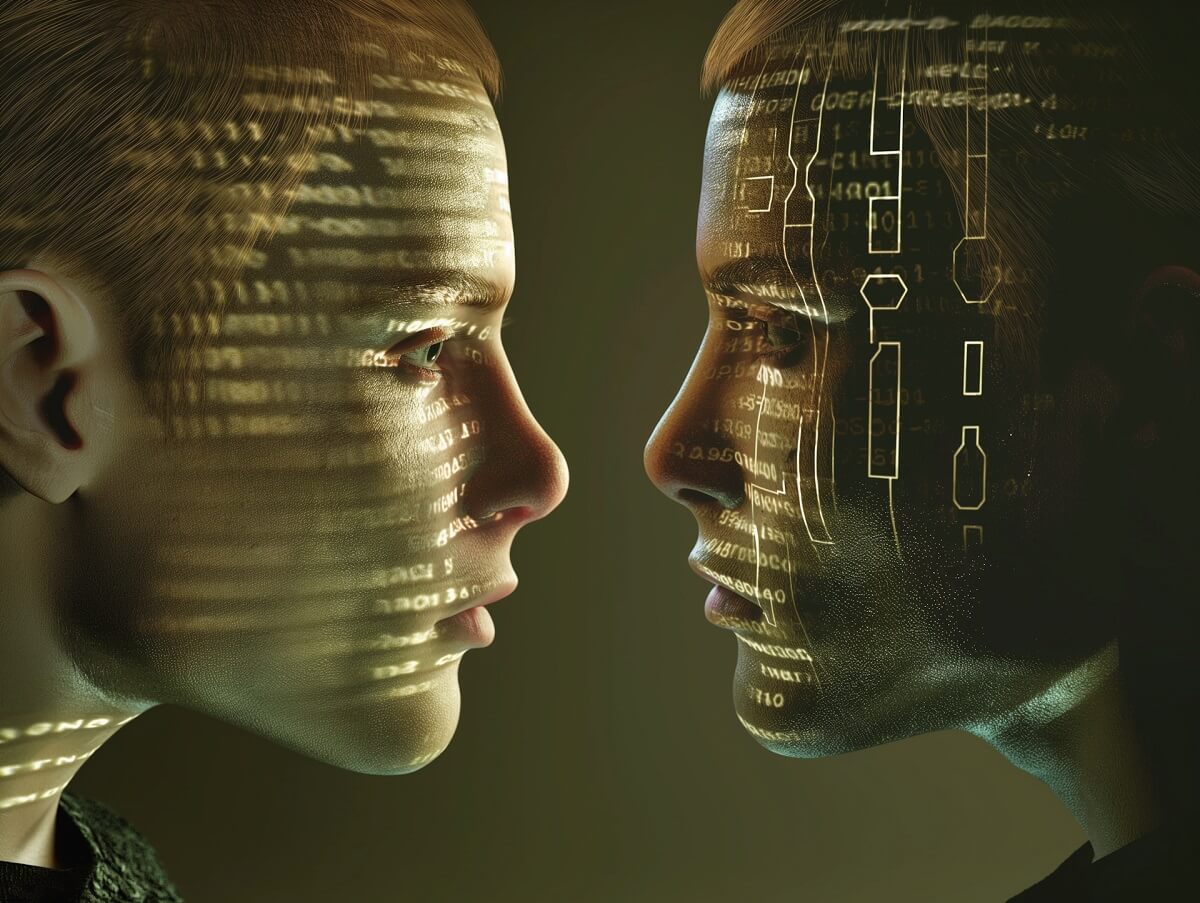
Ethics of Artificial Intelligence in Engineering Applications
Introduction
The rapid advancement of artificial intelligence (AI) in engineering has brought about revolutionary transformations across industries. While AI presents unprecedented opportunities, it also raises critical ethical concerns that need to be addressed. As engineers and developers increasingly integrate AI into critical systems—from autonomous vehicles to medical devices—the responsibility of ensuring ethical standards becomes paramount.
With AI systems now making decisions that affect human lives and societal structures, questions around responsibility, transparency, bias, and privacy have emerged. The lack of a unified ethical framework for AI in engineering applications has left many professionals grappling with how to implement these powerful technologies responsibly.
This article will delve into the core ethical issues surrounding AI in engineering applications, offering clear and actionable insights into how engineers can address these challenges. By understanding the implications of AI, engineers can ensure their work not only meets technical standards but also aligns with ethical principles.
The Responsibility and Accountability of AI in Engineering
One of the most pressing ethical concerns in AI applications is determining who is responsible for the decisions AI systems make. In engineering applications where safety, reliability, and human welfare are on the line, the question of accountability is vital. AI systems can autonomously make decisions based on large data sets, but when something goes wrong, who is held accountable?
AI is not a stand-alone entity; it operates under the control and guidance of engineers and developers. Engineers must ensure that the systems they create are not only functional but also ethical, meaning they must anticipate potential outcomes and take responsibility for their designs. Clear accountability frameworks must be established, where responsibility for an AI system’s behavior can be traced back to the humans who designed, deployed, and maintained it.
Transparency and Explainability in AI Models
AI systems, particularly those based on complex machine learning algorithms, are often referred to as "black boxes" due to their opaque nature. In engineering, where transparency is crucial, especially in sectors like healthcare, autonomous transportation, and public infrastructure, the inability to explain AI decision-making processes poses significant ethical risks.
Transparency in AI models is essential for building trust. Engineers need to ensure that the AI systems they develop can explain their decisions in a way that is understandable to humans. For example, in medical diagnostics, a doctor must be able to understand and verify the reasoning behind an AI's diagnosis before implementing any treatment plan. Explainability allows for informed decision-making and fosters trust between humans and machines.
Privacy and Data Security in AI-Driven Engineering
AI systems thrive on data, and in engineering applications, this often means collecting vast amounts of sensitive information. Whether it's personal health data in medical engineering or location data in autonomous vehicles, the ethical handling of this data is critical.
Data privacy must be a top priority for engineers working with AI. Strict data protection protocols should be enforced to ensure that AI systems do not inadvertently violate user privacy. Additionally, engineers must implement robust security measures to protect data from breaches, leaks, or unauthorized access. Ethical AI systems should respect user privacy while being transparent about what data is being collected and how it will be used.
Bias and Fairness in AI Systems
AI systems are only as good as the data they're trained on, and this can introduce bias into the systems, leading to unethical outcomes. For instance, facial recognition systems have been shown to perform poorly on individuals from certain racial or ethnic backgrounds due to biased training data. In engineering applications, such biases can lead to discrimination, inequality, and unfair treatment.
Addressing bias in AI is an ethical imperative for engineers. To build fair and unbiased systems, engineers must ensure that the data used to train AI models is representative and free from skewed patterns. Regular audits and testing should be conducted to identify and mitigate bias in AI systems. Ensuring fairness in AI applications not only improves the technology’s reliability but also fosters inclusivity.
Human-AI Collaboration in Engineering
In many engineering applications, AI systems work alongside human operators to make decisions. Whether it's in autonomous drones or advanced manufacturing robots, human-AI collaboration presents unique ethical challenges.
AI systems may outperform humans in certain tasks, but they should not replace human oversight, particularly in critical applications where safety is concerned. Engineers must strike the right balance between human and machine decision-making, ensuring that humans remain in control of AI systems, especially in high-stakes environments. Maintaining this balance ensures that AI serves as a tool to enhance human capabilities, rather than replacing human judgment entirely.
Regulatory Frameworks for Ethical AI Deployment
As AI technology continues to evolve, the need for regulatory frameworks that govern its ethical use becomes increasingly important. At present, there are few established global standards for ethical AI development, leaving many engineers uncertain about best practices.
Governments and industry bodies are beginning to develop regulations to address these gaps, but engineers must also take proactive steps to adhere to ethical guidelines in the absence of formal rules. Following frameworks such as ISO 26262 for automotive safety or IEEE’s Global Initiative on Ethics of Autonomous and Intelligent Systems can help ensure that ethical considerations are embedded in the design and deployment of AI systems in engineering.
Sustainability and Ethical Resource Use in AI
While AI can lead to more efficient processes, it also requires significant computational power, which has environmental implications. The energy consumption of large-scale AI models, especially in engineering simulations and design processes, can be significant.
Sustainability in AI development is an often-overlooked ethical consideration. Engineers must ensure that the benefits of AI applications are not overshadowed by the negative environmental impact of their energy use. Incorporating energy-efficient AI algorithms and using renewable energy sources in data centers are some ways to address these concerns. Ethical AI systems should not only be responsible in their outputs but also in their resource consumption.
The Future of AI Ethics in Engineering
As AI continues to permeate every aspect of engineering, the ethical challenges it presents will only grow more complex. Engineers will need to stay informed about evolving ethical standards, emerging technologies, and the societal implications of their work.
Ethical AI development is not just about following rules—it's about fostering a culture of responsibility, transparency, and fairness in every phase of the AI lifecycle. By adhering to ethical principles, engineers can help ensure that AI applications contribute positively to society without causing unintended harm.
Conclusion
The integration of AI in engineering applications offers exciting possibilities but also poses significant ethical challenges. Engineers must navigate issues of accountability, transparency, bias, privacy, human-AI collaboration, and sustainability to ensure that AI technologies are used responsibly.
The ethical challenges discussed in this article highlight the importance of building AI systems that are fair, explainable, and secure, ensuring they benefit society as a whole. As AI continues to evolve, engineers have a responsibility to develop solutions that not only solve technical problems but also address the moral and ethical implications of AI-driven technologies.
Artificial intelligence (AI)