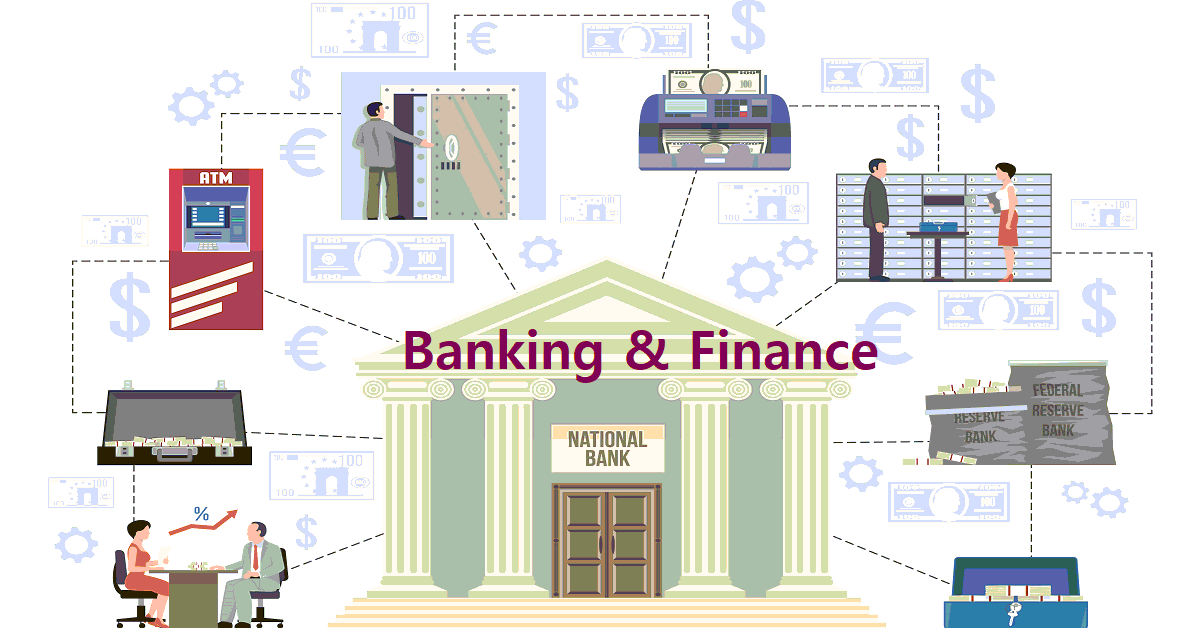
The Powerful Benefits of Data Analytics in Banking
Introduction
In the digital era, data has become the lifeblood of industries, and banking is no exception. The use of data analytics in banking has transformed the way financial institutions operate, offering unprecedented benefits and paving the way for a more efficient, customer-centric approach. This article delves into the profound impact of data analytics on the banking sector, highlighting its benefits, applications, and future trends.
The Emergence of Data Analytics in Banking
Data analytics, also known as banking analytics or financial data analysis, refers to the process of examining vast volumes of raw data to draw meaningful insights. These data-driven insights are then used to inform decision-making, enhance operational efficiency, and improve customer experiences in the banking sector.
Benefits of Data Analytics in the Banking Sector
Data analytics offers a myriad of benefits to the banking industry. Here are some key advantages:
-
Improved Risk Management: Data analytics allows banks to assess and manage risks more effectively. By analyzing historical data, banks can predict potential risks and take proactive measures to mitigate them.
-
Enhanced Fraud Detection and Prevention: With sophisticated analytical tools, banks can detect unusual patterns and anomalies that may indicate fraudulent activities. This not only helps in preventing fraud but also enhances the security of banking operations.
-
Better Customer Segmentation and Targeting: Data analytics enables banks to understand their customers' behaviors and preferences better, allowing for more precise segmentation and targeting.
-
Increased Operational Efficiency: By identifying inefficiencies and bottlenecks, data analytics can help banks streamline their operations and improve productivity.
-
Personalized Banking Experiences: With insights gleaned from data analytics, banks can offer personalized services and products, enhancing customer satisfaction and loyalty.
-
Informed Credit Scoring and Loan Underwriting: Data analytics can aid in assessing the creditworthiness of customers, leading to more accurate credit scoring and loan underwriting.
Examples of Data Analytics in Banking
Risk Management
One of the most significant applications of data analytics in banking is risk management. For instance, banks use predictive analytics to forecast potential loan defaults based on customers' credit history, income level, and other relevant factors. This helps banks make informed decisions and minimize the risk of bad debts.
Fraud Detection and Prevention
Data analytics plays a crucial role in detecting and preventing fraud. Banks use machine learning algorithms to analyze transaction data in real-time and identify suspicious activities. For instance, if a customer's card is used in two different locations within a short time, the system flags it as a potential fraud.
Customer Segmentation and Targeting
Banks use data analytics to segment their customers based on various parameters like age, income, spending habits, and more. This helps them tailor their marketing strategies and offer personalized services to different customer segments.
Operational Efficiency
Data analytics can help banks identify operational inefficiencies and areas for improvement. For instance, by analyzing transaction data, banks can identify peak hours and allocate resources accordingly to reduce waiting times and improve customer service.
Personalized Banking Experiences
With data analytics, banks can offer personalized banking experiences to their customers. For example, by analyzing a customer's transaction history, banks can recommend relevant products and services, enhancing customer engagement and loyalty.
Credit Scoring and Loan Underwriting
Data analytics plays a crucial role in credit scoring and loan underwriting. By analyzing a customer's financial history, banks can assess their creditworthiness and make informed lending decisions.
Challenges and Considerations in Implementing Data Analytics in Banking
While data analytics offers numerous benefits, implementing it in the banking sector comes with its own set of challenges. These include:
-
Data Privacy and Security: With the increasing use of data analytics, banks need to ensure the privacy and security of customer data. This requires robust data governance policies and practices.
-
Data Quality: The effectiveness of data analytics depends on the quality of data. Banks need to ensure that the data they use is accurate, complete, and up-to-date.
-
Regulatory Compliance: Banks need to comply with various regulations related to data usage and privacy. Non-compliance can result in hefty fines and reputational damage.
-
Skills and Expertise: Implementing data analytics requires specialized skills and expertise. Banks need to invest in training and development to equip their staff with the necessary skills.
Case Studies: Successful Data Analytics Initiatives in Banking
Case Study 1: JPMorgan Chase
JPMorgan Chase, one of the largest banks in the US, has been at the forefront of using data analytics. The bank uses machine learning algorithms to analyze customer data and offer personalized services. This has resulted in improved customer satisfaction and increased revenue.
Case Study 2: Wells Fargo
Wells Fargo uses data analytics for risk management. The bank uses predictive analytics to assess the risk of loan defaults, helping it make informed lending decisions and minimize bad debts.
Future Trends in Data Analytics for the Banking Industry
As we look towards the future, the role of data analytics in banking is set to become even more critical. Here are some trends to watch out for:
-
Artificial Intelligence and Machine Learning: AI and ML will play a pivotal role in data analytics, offering more accurate predictions and insights.
-
Real-Time Analytics: With the advent of real-time analytics, banks will be able to analyze data in real-time and make instant decisions.
-
Predictive Analytics: The use of predictive analytics will increase, helping banks forecast future trends and make proactive decisions.
-
Data Visualization: Data visualization tools will become more sophisticated, making it easier for banks to understand and interpret data.
Conclusion
Data analytics has revolutionized the banking industry, offering numerous benefits and transforming the way banks operate. As we move towards a more data-driven future, the role of data analytics in banking is set to become even more critical. By embracing data analytics, banks can enhance their operations, improve customer experiences, and drive informed decision-making.
"Data is the new oil. It’s valuable, but if unrefined it cannot really be used. It has to be changed into gas, plastic, chemicals, etc to create a valuable entity that drives profitable activity; so must data be broken down, analyzed for it to have value." - Clive Humby, UK Mathematician and architect of Tesco's Clubcard, 2006.
In the context of banking, this quote perfectly encapsulates the power and potential of data analytics. As we've seen, when properly refined and analyzed, data can drive profitable activity, inform decision-making, and ultimately, transform the banking industry.
Banking and Finance