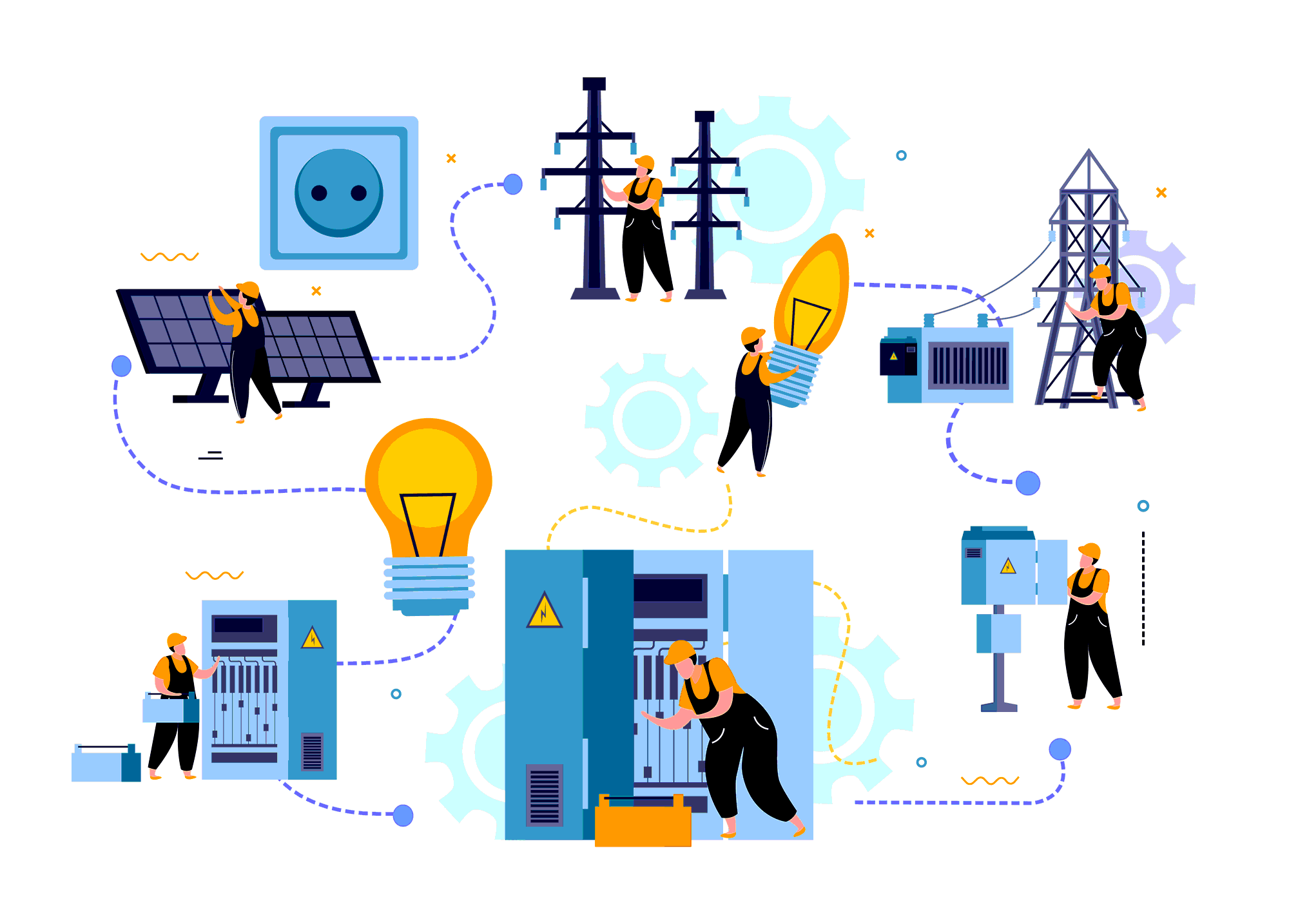
Electric Load Forecasting: Techniques for Accurate Predictions
Electric load forecasting is a crucial process in the energy and utility industries that involves predicting the amount of electricity that will be consumed by consumers over a certain period of time. This information is necessary for utility companies to plan and operate their power grids efficiently, ensuring that they have enough supply to meet demand. Inaccurate electric load forecasting can result in blackouts, brownouts, or oversupply of electricity, which can be costly for both utility companies and consumers. In this article, we will explore the most effective techniques for predicting electric loads accurately.
Overview of Electric Load Forecasting
Electric load forecasting involves analyzing historical data and using it to make predictions about future electricity demand. There are different methods for electric load forecasting, including statistical models, machine learning, and hybrid models. The accuracy of load forecasting depends on various factors, such as the quality of data, the chosen forecasting method, and the complexity of the energy system.
Methods for Electric Load Forecasting
Statistical Models: Statistical models are widely used for electric load forecasting and involve analyzing historical data to identify patterns and trends. Common statistical models used for load forecasting include time-series analysis, regression analysis, and exponential smoothing. These models are relatively simple to implement and interpret, making them a popular choice for utilities with limited resources. However, they may not perform well when faced with sudden changes in demand or when dealing with highly volatile data.
Machine Learning: Machine learning involves using algorithms to analyze large datasets and identify patterns that can be used for predictions. Machine learning models can handle complex data sets, including non-linear relationships and interactions between variables. They can also adjust to changing patterns in data, making them suitable for forecasting highly volatile demand. Machine learning models have been found to outperform statistical models in some cases, as demonstrated by a study by the National Renewable Energy Laboratory.
Hybrid Models: Hybrid models combine statistical models and machine learning algorithms to take advantage of the strengths of each approach. For example, the California Independent System Operator (CAISO) uses a hybrid approach for electric load forecasting, combining statistical models and machine learning algorithms. Hybrid models can be more accurate than either method used alone, but they can also be more complex to develop and implement.
Advantages and Disadvantages of Each Method
Each electric load forecasting method has its advantages and disadvantages. Statistical models are easy to interpret and implement, but they may not perform well in highly volatile situations. Machine learning models can handle complex datasets and adjust to changing patterns, but they require significant computing power and may be more challenging to interpret. Hybrid models can offer the best of both worlds, but they may require more resources and expertise to develop and implement.
Latest Advancements and Research in Electric Load Forecasting
The electric load forecasting market is expected to grow from $2.9 billion in 2020 to $4.3 billion by 2025, according to a report by MarketsandMarkets. This growth is due to the increasing demand for renewable energy, the need for efficient energy management, and the development of smart grid technology. Recent research has focused on improving the accuracy of electric load forecasting models, including the use of big data analytics, deep learning, and ensemble models.
Techniques for Improving the Accuracy of Electric Load Forecasting Models
To improve the accuracy of electric load forecasting models, utilities can take several steps, including:
- Using high-quality data and regularly updating it to reflect changing patterns
- Using multiple forecasting methods and comparing their results to identify the most accurate approach
- Incorporating external factors such as weather forecasts and economic indicators that can impact electricity demand
- Using ensemble models that combine the results of multiple forecasting methods to increase accuracy
- Implementing real-time monitoring and feedback mechanisms to adjust forecasts as needed
Case Studies/Examples of Successful Electric LoadĀ Forecasting:
Case Study 1: Google's DeepMind AI for Electric Load Forecasting
In 2018, Google's DeepMind team introduced an artificial intelligence (AI) system for electric load forecasting. The system uses deep learning algorithms to analyze historical data and make predictions about future electric load. According to Google, the system has improved the accuracy of electric load forecasting by 40%, resulting in significant cost savings for the company.
Case Study 2: Duke Energy's Hybrid Approach to Electric Load Forecasting
Duke Energy, one of the largest electric power holding companies in the United States, uses a hybrid approach for electric load forecasting. The company combines statistical models with machine learning algorithms to make predictions about future electric demand. According to Duke Energy, the hybrid approach has improved the accuracy of electric load forecasting by up to 15%.
These case studies demonstrate the potential benefits of using advanced techniques for electric load forecasting. By combining historical data with machine learning algorithms, companies can improve the accuracy of their predictions and avoid costly over- or under-provisioning of power.
Conclusion:
Electric load forecasting is a critical function for the energy and utility industries. Accurate predictions of electric demand enable companies to optimize power generation and transmission, reducing costs and improving reliability. There are several methods for electric load forecasting, including statistical models, machine learning, and hybrid models.
Each method has its advantages and disadvantages, and the choice of method depends on the specific needs of the company. However, recent advancements in machine learning and artificial intelligence have opened up new possibilities for electric load forecasting, resulting in significant improvements in accuracy and cost savings.
To improve the accuracy of electric load forecasting models, companies can take several steps, including collecting high-quality data, using advanced algorithms, and integrating external factors such as weather patterns and renewable energy sources.
By implementing these techniques, companies can achieve more accurate and reliable predictions of electric demand, resulting in improved efficiency and cost savings. As the demand for electric load forecasting continues to grow, companies that adopt advanced techniques and technologies will be better positioned to succeed in the competitive energy and utility markets.
Electrical Engineering