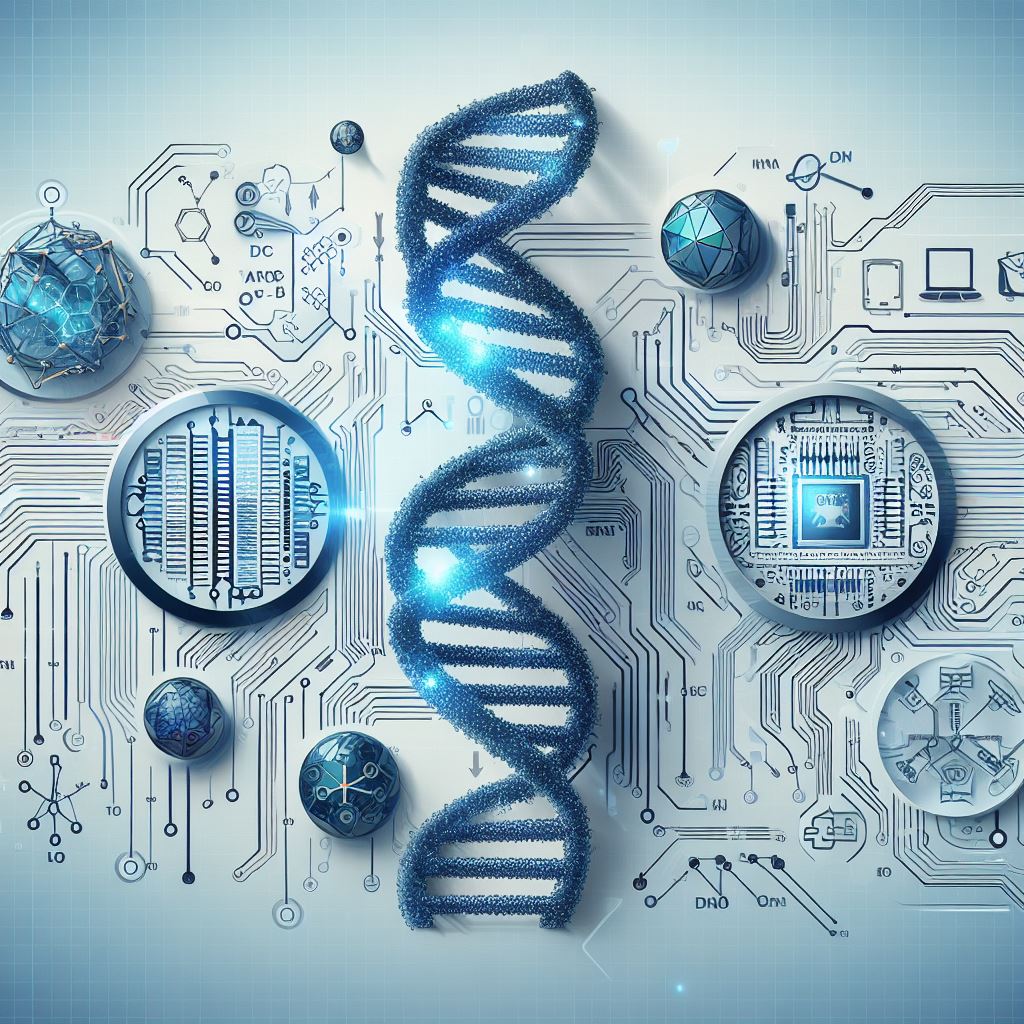
Computational Biology: Unraveling Complex Biological Systems Through Advanced Algorithms
Introduction
In the modern era, where biology meets technology, we're gifted with the science of Computational Biology. This evolving discipline uses advanced algorithms to decode and understand the intricate biological systems that have eluded mankind for ages.
Definition and Overview of Computational Biology
Computational biology, often used interchangeably with bioinformatics and systems biology, is the application of computer science and mathematical models to understand biological processes. In essence, it provides a digital lens through which we can explore and study biology.
The Interdisciplinary Nature of Computational Biology
Computational biology is truly a melting pot of disciplines. At its core, it involves:
- Biology: The study of life and living organisms.
- Computer Science: Utilizing computational approaches to solve biological problems.
- Mathematics: Using statistical models to analyze and predict biological phenomena.
This unique blend ensures a holistic approach to understanding and solving complex biological challenges.
Applications of Computational Biology
1. Medicine
The applications of computational biology in medicine are vast:
- Personalized medicine, tailoring treatments based on individual genetic makeup.
- Predicting disease outbreaks using data-driven models.
- Understanding the genetic basis of diseases.
2. Genomics
Computational methods in genomics play a pivotal role in:
- Sequencing and annotating genomes.
- Identifying genes and predicting their functions.
- Understanding the evolutionary aspects of genes and genomes.
3. Drug Discovery
Integrative computational biology for drug discovery has changed the way pharmaceutical research operates:
- Identifying potential drug targets using computational methods.
- Predicting how different drugs interact with their target proteins.
- Enhancing drug design through molecular modeling.
The Role of Machine Learning in Computational Biology
Machine learning, a subset of artificial intelligence, has found its niche in computational biology. The role of machine learning in computational biology revolves around:
- Analyzing large sets of genetic data.
- Predicting protein structures.
- Understanding genetic variations and their implications on health.
For instance, Google's DeepMind developed AlphaFold, a system that predicts protein structures with remarkable accuracy. This breakthrough has immense potential implications for drug discovery and understanding diseases at a molecular level.
Challenges in Computational Biology
Despite its promises, computational biology isn't without challenges:
- Data Overload: The sheer volume of biological data generated poses storage and analysis challenges.
- Complexity of Biological Systems: Biological processes are often non-linear and intricate, making modeling them a daunting task.
- Integration of Data: Different biological studies often use varied data types, integrating them for a unified understanding can be challenging.
The Future of Computational Biology
In the era of big data and machine learning, computational biology is poised to become even more pivotal. We can foresee:
- Enhanced personalized medicine driven by data analytics.
- Integrative models that combine genomics, proteomics, and metabolomics for a holistic understanding of biological systems.
- Real-time disease outbreak prediction using advanced computational models.
Conclusion
As quoted by Dr. Pavel Pevzner in his book "Bioinformatics Algorithms: An Active Learning Approach," "The future of biology is computational." Computational biology, with its applications, challenges, and future prospects, is revolutionizing our understanding of biology, healthcare, and drug discovery. For those on the intersection of biology and technology, it's an exciting journey ahead.
References:
- Durbin, R., Eddy, S., Krogh, A., & Mitchison, G. (1998). Biological Sequence Analysis.
- Compeau, P., & Pevzner, P. (2015). Bioinformatics Algorithms: An Active Learning Approach.
- Journal of Computational Biology.
- Proceedings from the International Society for Computational Biology (ISCB).